First Confirmed Sessions
Machine Learning Beyond Classification and Regression: Predicting Assembly Plans for New 3D Product Designs at Daimler Trucks
Most of today’s machine learning tasks deal with predicting atomic values. In Classification, for each object a class is predicted, i.e. a category assigned. In Regression, for each object a numerical value is predicted. Both are simple predictions in the sense that they only predict a single value per object. Most machine learning algorithms are also simple in the sense that they can only handle simple linear feature vectors. We propose a complex problem: Given complex hierarchical 3D designs of new products, predict assembly times and automatically generate assembly plans, i.e. sequences pf assembly steps to manufacture these products. Our solution was validated by predicting assembly plans for new truck engine components at Daimler Trucks. In this case study we describe how to use machine learning to automatically predict assembly times and assembly plans for new complex product designs. This enables car makers and other manufacturers to accelerate the product design and assembly planning process, increasing the agility of the company and reducing the initial costs for new products.
Automated Evaluation of Machine Wear and Tear in Manufacturing
Wear and tear of our customer’s production machines causes sudden failures and unexpected down times which often results in heavy losses. To increase machine availability our customer offers a diagnosis service based on vibration measurements. However, till now the manual vibration evaluation by machine experts represents a bottleneck of the service and prevents regular monitoring of machines. To address this, we developed a data analytics tool integrating domain knowledge and machine learning algorithms to quantify machine tear. Due to the automated evaluation our customer can offer a new diagnosis service to continuously monitor machine wear and thus reduce machine downtime.
Try Fast, Fail Fast & Adjust Fast: HP’s Philosophy Towards its Industry 4.0 Efforts
Industry 4.0, though a buzzword, can be maddening to lots of companies. We believe HP Inc’s Supplies Organization experience is no different. One of the key foundation that has enabled HP to achieve success is the philosophy of “Try Fast, Fail Fast & Adjust Fast”. This philosophy has enable us to cope with uncertainties and unknowns that comes with innovation. This presentation will share some of the critical instances of this philosophy along our journey towards developing Manufacturing Analytics in HP. It will show case tries, failures and most importantly, how we regrouped, learn & adjust to achieve success!
Preventing Big Balls of Fire at INEOS
INEOS is one of the world’s largest manufacturers of chemicals and oil products. About every two years in the Cologne plant, safety mechanisms in Polyethylene production are triggered due to a so-called decomposition event, leading to a large “ball of fire” above the plant. These events are within operating parameters of the plant, but naturally lead to significant reputational and financial costs for INEOS. Even though decompositions have been studied for decades, there is so far no reliable predictor for them. Therefore, INEOS and ONE LOGIC attempted a novel, machine-learning based approach to predict and thereby prevent these dramatic events.
Happy Cow – Happy Farmer! A Commercial IoT Use Case in Massive Farming Data for Better Animal Health
“Cows wearing fitness tracker” sounds innovative, but its common animal monitoring since decades in farming. But since, available data in farming has grown exponentially. These days a modern farm equals rather a production facility when it comes to the use of IoT. Hundreds of sensors continuously monitor a cows life over multiple automated sources as i.e. milking robots, feeding and drinking robots, sensors for heart- & activity rate, milk-quality, fertilization, weight, barn atmosphere, etc. In the presented use case all daily data has been collected over more than 3 years and hundreds of cows to build analytics capabilities to predict rare animal diseases. The objective: releasing animal pain and optimizing milk productivity and quality. This presentation will provide insights into the project and will provide practical learnings when it comes to balance interests between scientific research and commercial interests as well as expert-dynamics when traditional statistician meet machine learning engineers to serve a higher well.
Data Science Methods at Work in the Vodafone Network
Communication networks provide large data sets which are perfectly suited for data science methods. By employing these advanced analytics techniques, customer experience can be simulated, predicted and improved. Service quality can be enhanced and processes are automated. Examples for predictive analytics at work in the Vodafone Germany networks will be shown: from customer experience simulations and predictions as input for network capacity planning towards network problem predictions via time series. This will also include machine learning triggering real-time actions, and thus digitalizing and automating the network maintenance processes.
Realizing the True Value in Your Data: Data-Drivenness Assessment in Logistics & Supply Chain
During our work, many companies have come to us looking to implement a specific tool or new analyses to improve business performance. After collecting data and building out models, the initiative falls apart because the analytical wherewithal is not in place across the organization or department. Stepping back from these problems, we’ve identified four areas that firms need to constantly asses to ensure they are leveraging their data to its maximum potential: Data Strategy; Data Culture; Data Management & Architecture; Data Analysis, Visualization & Implementation.
During this presentation Lawrence will discuss each of these areas in depth, including our methodology for assessing performance, common pitfalls we’ve seen across industries, and key learnings through many case studies within the logistics and supply chain space. Within each area, we have developed a thorough methodology for assessing a firm’s performance and identifying opportunities for improvement.
Engineering Meta-Optimisation Techniques in Logistics
Your customers buy products online and it’s time to pack the items for shipping and deliver them to customers. There’s tons of applicable process optimisation, e.g.: How many people to pack it? What is the best warehouse layout to maximise productivity? How many vans are needed for deliveries? How many deliveries per time slot? But the optimisation itself can also be optimised, to make it more efficient, precise or robust. We will look at engineering methods used for optimising the logistics optimisation (meta-optimisation) and show how clever engineering helps delivering efficient service.
Data-driven Plant Factory: Sensor Data, Machine Learning and Optimization for Indoor Farming at Scale
With more of the world’s population moving to cities, indoor farms that grow crops near urban areas in a way that is efficient with space and other resources may be an important way forward. Dramatic improvements in crop yield and operational efficiency can be made by using data (collected for instance by sensors or cameras) to build an increasingly smart plant factory. This plant factory allows us to control the environment for crops to grow more, better-tasting crops in a way that is reproducible. By iteratively automating processes in the farm, we ensure that this can be done in a way that scales to the levels of production needed. Creating data products that allow us to automate does come with some challenges. One such challenge is balancing the need to develop temporary solutions that provide value early with the risk of enshrining existing workarounds and creating tech debt. In this case study, I will discuss the iterative process we use to automate and improve decisions around the farm, with a focus on operations.
Blockchain-backed analytics: Adding trust to data-driven projects
Blockchain-backed analytics (BBA) is a scientific concept to transparently document the lineage and linkage of the three major components of a data-driven project: data, model & result. The approach enables stakeholders of data science projects to track and trace data, trained/applied models and modelling results without the need of trust validation of escrow systems or any other third party.
This talk covers the theoretical concept of BBA and showcases a generic appliance. Participants will learn how to design blockchain-backed analytics solution for e.g. situations in which industrial partners share and distribute data, models and results in an untrusted setting.
Agile for Analytics – Best Practices & Strategy along the Road from a Business Idea to a Data-Driven Analytics Product or Service with Business Impact
For data-driven analytics products, the ideal setup that leads to a successful product is the trifecta of business value, analytics (data science), and information technology (IT). We follow lean and agile principles and practices from Design Thinking, Lean Startup, Scrum, Kanban, and the Team-Data-Science-Process by Microsoft. We present best practices over different product development phases and life cycles from ideation, Value Proposition (VP) Design, hackathons, minimum viable product (MVP) development, and finally to software product development utilizing strategic tools: VP, Lean Canvas and/or Business Model Canvas, Logic Model and/or Impact Map, and metrics derived from the MVP/product and its development.
Beyond the Data Lab: Advanced Analytics at Continental Tire
For global companies like Continental, advanced analytics and artificial intelligence promise numerous applications and significant opportunities. But how can these be seized quickly by global organizations? Continental’s Tire division achieved this by working towards three targets in parallel: first, quickly building the right infrastructure that uses containerization and automated machine learning; second, quick wins’ by bringing first use cases into production, starting with demand forecasting, supply chain and IoT; third, strengthening agile principles to enhance how both individual analytics projects and the overall organization work. Our talk will present in depth our infrastructure, use cases, and agile approaches.
*/ ?>
Predictive Analytics World for Industry 4.0 - Munich - Day 1 - Monday, 6th May 2019
Ann Winblad called data at CNBC "the new oil". Since then, there haven’t been too many data-driven business models outside advertising–and certainly not in the brick and mortar industries.
On the other hand, politicians, interest groups, activists, and lobbyists outdo each other with new and often contradicting ideas on how to regulate and govern data.So far mostly personal data was in focus (cf. GDPR, New California Consumer Privacy Act). But just recently in Germany SPD’s Andrea Nahles demands mandatory data publishing for all non-person related (i.e., machine) data, putting EU’s industry at risk.
Now, how can data & analytics (or AI) lead to new sources of wealth? What are the advantages of startups and what is the situation for incumbent companies? What role models and good examples across industries are there? And could there be a European data platform at eye level to the US or China?
Learn how Edge Analytics can be critical to the success of your Predictive Analytics project:
- Transform raw machine data in the Edge in real-time
- Reduce cost of bandwidth, cloud and data analytics tools
- Go deeper without increasing the data volume
- Implement anomaly detection models in the Edge
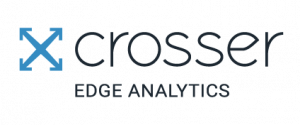
For global companies like Continental, advanced analytics and artificial intelligence promise numerous applications and significant opportunities. But how can these be seized quickly by global organisations? Continental’s Tire division achieved this by working towards three targets in parallel: first, quickly building the right infrastructure that uses containerisation and automated machine learning; second, quick wins’ by bringing first use cases into production, starting with demand forecasting, supply chain and IoT; third, strengthening agile principles to enhance how both individual analytics projects and the overall organisation work. Our talk will present in depth our infrastructure, use cases, and agile approaches.
Time plays a major role in industrial manufacturing. Machine tools are constantly optimized to maximize value-adding activities. An intelligent manufacturing assistant for machine tools has been developed within Schaeffler's digitalization department, which allows an individual Overall Equipment Effectiveness (OEE) increase in machines. Through a modular architecture a self-learning AI concept with high transferability was implemented. It allows to analyze wearing components dynamically based on sensor data and gives recommendations for actions on the machine.
Linear, Machine Learning and Probabilistic models are often used in the predictive analytics. Each of them has its pros and cons for different industrial and business problems. Linear models make it possible to extrapolate forecasting, study impact of external factors but does not allow us to capture nonlinear complicated patterns in the data. Machine learning models can find a complicated pattern but only in the stationary data, at the same time these models require a lot of historical data for training to get sufficient accuracy. Probabilistic models based on the Bayesian inference can take into account expert opinion via prior distributions for parameters and can be used for different kinds of risk assessments. In the speech, I am going to consider the use of these models and their combinations in different use cases. One type of use case is numeric regression for time series forecasting, another one is logistic regression in manufacturing failure detection problems. I will also consider multilevel predictive ensembles of models based on the bagging and stacking approaches.
Most of today's machine learning tasks deal with predicting atomic values. In Classification, for each object a class is predicted, i.e. a category assigned. In Regression, for each object a numerical value is predicted. Both are simple predictions in the sense that they only predict a single value per object. Most machine learning algorithms are also simple in the sense that they can only handle simple linear feature vectors. We propose a complex problem: Given complex hierarchical 3D designs of new products, predict assembly times and automatically generate assembly plans, i.e. sequences pf assembly steps to manufacture these products. Our solution was validated by predicting assembly plans for new truck engine components at Daimler Trucks. In this case study we describe how to use machine learning to automatically predict assembly times and assembly plans for new complex product designs. This enables car makers and other manufacturers to accelerate the product design and assembly planning process, increasing the agility of the company and reducing the initial costs for new products.
Using finite element method (FEM), physical behaviors of a car are simulated in crash use cases. The parametrized FEM is used in simulation runs which consumes a significant amount of time resources because the causalities of input parameters and results of the simulation is not transparent and highly complex. For reducing simulation runs, most promising FE parametrizations shall be made transparent using results from former simulation runs. This data needs to pre-processed for feature extraction and consequently can be used as input training data for the prediction model. The results of the feature extraction makes coherences in FE parametrization transparent.
In this presentation we will demonstrate approaches that can be used for the development, validation, and deployment of predictive models within regulated environments. In particular, we will describe a open-source predictive analytics platform we are building for use within the healthcare setting. We will discuss the approaches used for validating data and models, ongoing performance assessment, and methods used to scale and audit predictive analytic pipelines. We will work through real-world uses cases based on this platform, including predictive models for pharmacogenomic screening and medical image analysis.
Deep learning can be used to replace humans for visually inspecting things like cars and electrical cabinets. When you rent a car, a person walks around the vehicle with a clipboard and writes down (on paper!) a list of any damage on the vehicle - before and after you rent it. As we move to a decentralized sharing economy, this process will need to change and deep learning can be used to do automatic visual damage inspections on vehicles. Similarly, work done on large infrastructure projects is manually inspected (with a delay) by humans leading to inefficiencies and safety issues that could be prevented using real-time error detection powered by deep learning. This talk will present work we have done on visual inspections with a large car manufacturer and a multinational infrastructure service provider.
Labelled data is a mandatory prerequisite for regression and classification tasks. However, labeling data can be expensive and sometimes it is even unfeasible. What to do when labels are scarce? In this talk I present a semi-supervised approach to detecting defects in images of industrial parts that was developed for Miba. The content ranges from the problems and limitations that arise from little labelled data to how we concretely solved it in the context of the project following a multi-level, semi-supervised approach combining deep learning and traditional machine learning techniques.
Industry 4.0 is driven by digital revolution and digital twins. With the availability of better data, AI techniques could be used to better predict when the machines needs repair/maintenance and also compute the remaining-life of the machine. But which technique to pick? Should we start with a Machine Learning or a Deep Learning technique? Which technique? There are around 5000 known statistical techniques that could be used to detect anomalous behavior of machines or vehicles. k-NN, LOF, INFLO, CBLOF, uCBLOF, ocSVM, rPCA, etc. are some of them. Deep Learning techniques, independently and in conjunction with machine learning techniques, are further improving the quality of predictions.
From the start Predictive Analytics World has been the place to discuss and share our common problems. These are your people – they understand your situation. Often rated the best session of all, sharing your problems with like-minded professionals is your path to answers and a stronger professional network.
There will be two discussion rounds of 30 minutes each. So choose your two most burning topics and discuss with your colleagues:
- Big Corporate vs. Lean Startup: What Can We Learn From Each Other? (with Dr. Andreas Braun)
- Manufacturing Analytics: What else is there besides Predictive Maintenance? (with Richard Lim)
- Jobs below the API: Can we automate with workers' dignity in mind? (with Dr. Marianne Hoogeveen)
From the start Predictive Analytics World has been the place to discuss and share our common problems. These are your people – they understand your situation. Often rated the best session of all, sharing your problems with like-minded professionals is your path to answers and a stronger professional network.
There will be two discussion rounds of 30 minutes each. So choose your two most burning topics and discuss with your colleagues:
- How to Convince Your Boss to Put a Model Into Production (with SK Reddy)
- Automating Predictive Analytics: an Unrealistic Vision or a Ready-to-Use Solution? (with Dr. Sandro Saitta)
- What’s the Future of Industry Analytics: Deep Learning, Quantum Computing, Blockchain or What Else? (with Frank Pörschmann)
Russmann as a licensed partner of AVIS provides rental cars and vans, as well as vehicle sharing and leasing solutions had a need for improving the cost-effectiveness of their business and increasing the fleet utilization rate. Leveraging data previously disconnected, we achieved those objectives. We present a solution based on modern forecasting techniques including machine learning algorithms, classical statistical methods, and optimization approaches that allowed managers make more effective data-driven transfer decisions and reduce car transfer costs as a result. Due to implementation of this solution, we eliminated the need for station managers to distribute vehicles manually and decreased the costs on new fleet purchases due to more accurate demand prediction. Boosted fleet utilization rate and reduced transfer costs helped the company to increase its profitability. This track will demonstrate the practical approaches to demand forecasting and advanced planning. I will give you an overview on the basics of smart capacity management and how you can use a post-factor analysis to understand business needs better.
This talks explains how Homag build the machine learning based operator assistance system intelliGuide for panel dividing saws. It walks you through the approach we took, the challenges we faced and the lessons we learned from it. intelliGuide is an mechatronic operator assistance system that allows the machine to see what the operator is intending to do and that warns him in case of an error or, if possible, dynamically adjust the machine processes to the current situation. The system is sold since 2017 and used by customers around the world.
In this deep dive, Valon presents a one shot learning novel approach for recognizing actions and detecting damages in industry. Action recognition is being applied everywhere, from autonomous driving to patient monitoring, whereas damage detection in the industry is mainly completed manually or semi-automatic. Current approaches require tens of hundreds of video samples for training models to recognize a specific action and tens of labeled images to detect damages. In the talk, Valon briefly discusses the algorithm and focuses on presenting the cases studies from the industry.
Predictive Analytics World for Industry 4.0 - Munich - Day 2 - Tuesday, 7th May 2019
Industry 4.0, though a buzzword, can be maddening to lots of companies. We believe HP Inc's Supplies Organization experience is no different. One of the key foundation that has enabled HP to achieve success is the philosophy of “Try Fast, Fail Fast & Adjust Fast”. This philosophy has enable us to cope with uncertainties and unknowns that comes with innovation. This presentation will share some of the critical instances of this philosophy along our journey towards developing Manufacturing Analytics in HP. It will show case tries, failures and most importantly, how we regrouped, learn & adjust to achieve success!
Be prepared to hear what is hot in AI today — and you will gain wider insight intoWhere From, Why Now, and especially To Whom Today!

Wear and tear of our customer’s production machines causes sudden failures and unexpected down times which often results in heavy losses. To increase machine availability our customer offers a diagnosis service based on vibration measurements. However, till now the manual vibration evaluation by machine experts represents a bottleneck of the service and prevents regular monitoring of machines. To address this, we developed a data analytics tool integrating domain knowledge and machine learning algorithms to quantify machine tear. Due to the automated evaluation our customer can offer a new diagnosis service to continuously monitor machine wear and thus reduce machine downtime.
Physical process or machinery control is in the main hard engineering work. Control hierarchy, such as Proportional-Integral-Derivative (PID) and Model Predictive Control (MPC) setup and tuning is slow, even in simulators. And the control target never stays the same, due to e.g. ageing and environmental conditions resulting in suboptimal efficiency, performance and machinery lifetime. Machinery builders have the problem for every new model or configuration of e.g. a mining machine or part. Often there are no matching parallel simulators for a specific model, meaning the R&D is done with the physical prototype, with real-time constraints. Our neural MPC machine learning solution works by creating a world model from data by running the actual machinery with rudimentary control and sensors. After these initial runs, the neural MPC and online learning keep the system adaptive to any changes. Integration time is measured in weeks for the interface and control targets and collecting the initial data to get a functioning control system for a machine.
Trains passing through a switch produce vibrations on the track, which can help to diagnose the health of the bed track and the switch itself. For that purpose it is useful to control for train type and speed. The vibrations contain a train fingerprint, which can be identified with help of deep learning and other machine learning classifiers. The purpose of this talk is to provide a walkthrough over the whole data pipeline, from preprocessing and classical signal processing, over the individual tier 1 deep learning models, and onto the aggregating tier 2 model. Moreover, the data originates from an evolving dynamical system, which requires that the classifier be a part of a continuous learning process in a semi-supervised manner to update the training set. This semi-supervised training will also be discussed in detail. Many of the techniques will be familiar from speech-to-text applications, but they needed to be updated for the particular requirements of this problem.
Bayer partnered with Accenture to develop a set of machine learning algorithms on top of the cross-functional BI platform to predict the probability of default for individual sales products along the supply chain. In an often alert-based planning approach, this model provides the production planner a systematic view on the main risks, their likelihood and the underlying stock-out drivers. The planner is thus enabled to initiate mitigation measures early to prevent potential stock-outs. Essential part of the solution was connecting various data sets along the supply chain and balancing the trade-off between model complexity and interpretability tailored to the user.
Over stocking and out of stock situations in retail stores are major issues faced by FMCG companies. Ordered quantity for products is mostly based on informal assessment of store’s requirements by sales representatives done manually. This generally results in over supply or under supply of products. Our suggested order model helps FMCG companies to optimally predict order quantities for every store / product combination. The model uses ensemble of decision trees along with deep learning based feature embedding resulting in highly accurate predictions (> 80%). We have successfully developed the solution for major Indian FMCG companies like P&G (India), Id Fresh, and Parle. This presentation will showcase working of the algorithm and its outcome on different real world datasets.
In recent times, we have been able to cram more and more computational power into the chips. However, by 2020, silicone chips will not be able to support ‘Moore’s law’ anymore, and we will have to step off our beaten track for something entirely new. As the demand grows, we will have to replace traditional computing means with technologies that are more powerful and advanced. In May 2016, IBM launched its IBM Q, an industry-first initiative to build commercially available universal quantum computers for business and science. In this talk, we will explore the principal differences between classical and quantum computer programming. You will get the answers on how a quantum computer works and how to perform operations on it. Also, we will discuss Quantum ML algorithms and IBM QX as the crucial step towards the development of quantum computers.
How to add value to your customers and on the same time reduce costs and become more efficient with predictive analytics within the supply chain of a large smart factory solution provider? The topic of this use case presentation shows how Cognitive Business Robotics improves the predictive inventory management at Bossard, increases the productivity and lowers costs by predicting what material is needed when at which customer in a B2B scenario. The learning is on how to combine Robotic Process Automation with AI and Human in the Loop.
Downtime is not an option for your clients as it was for our client Hobart Services — repair technicians visiting remote locations must be successful on their first visit. How can you ensure you are carrying the right parts? Can we learn that from historical data? Technician notes possess years of complaint/cause/correction data that provide solutions when correlated with IoT data. Extracting these requires highly specialized NLP and machine learning models adapted to repair and maintenance — but allows you to empower field dispatchers with intelligent diagnosis and technicians with guided repair, reducing overall servicing costs and improving first call completion metrics. Attendees will learn enterprise AI techniques in the realm of repair and predictive maintenance and how to make use of noisy historical repair data in predictive analytics.
During our work, many companies have come to us looking to implement a specific tool or new analyses to improve business performance. After collecting data and building out models, the initiative falls apart because the analytical wherewithal is not in place across the organisation or department. Stepping back from these problems, we’ve identified four areas that firms need to constantly asses to ensure they are leveraging their data to its maximum potential: Data Strategy; Data Culture; Data Management & Architecture; Data Analysis, Visualisation & Implementation. During this presentation Lawrence will discuss each of these areas in depth, including our methodology for assessing performance, common pitfalls we’ve seen across industries, and key learnings through many case studies within the logistics and supply chain space. Within each area, we have developed a thorough methodology for assessing a firm’s performance and identifying opportunities for improvement.
The Deutsche Bahn invests heavily in energy efficiency. To that end, the most important drivers of energy consumption are identified and appropriate measures for increased efficiency are deduced. For planning purposes, it is furthermore necessary to predict energy consumption as accurately as possible. The main data source for forecasts are the remote energy consumption readings taken from the so called TEMA boxes installed on all trains. These data are then enriched using many additional data sources, for example on train schedules, train track topography, properties of traction-units, and on weather.
Communication networks provide large data sets which are perfectly suited for data science methods. By employing these advanced analytics techniques, customer experience can be simulated, predicted and improved. Service quality can be enhanced and processes are automated. Examples for predictive analytics at work in the Vodafone Germany networks will be shown: from customer experience simulations and predictions as input for network capacity planning towards network problem predictions via time series. This will also include machine learning triggering real-time actions, and thus digitalizing and automating the network maintenance processes.
Your customers buy products online and it's time to pack the items for shipping and deliver them to customers. There's tons of applicable process optimisation, e.g.: How many people to pack it? What is the best warehouse layout to maximise productivity? How many vans are needed for deliveries? How many deliveries per time slot? But the optimisation itself can also be optimised, to make it more efficient, precise or robust. We will look at engineering methods used for optimising the logistics optimisation (meta-optimisation) and show how clever engineering helps delivering efficient service.
Cows wearing fitness tracker” sounds innovative, but its common animal monitoring since decades in farming. But since, available data in farming has grown exponentially. These days a modern farm equals rather a production facility when it comes to the use of IoT. Hundreds of sensors continuously monitor a cows life over multiple automated sources as i.e. milking robots, feeding and drinking robots, sensors for heart- & activity rate, milk-quality, fertilisation, weight, barn atmosphere, etc. In the presented use case all daily data has been collected over more than 3 years and hundreds of cows to build analytics capabilities to predict rare animal diseases. The objective: releasing animal pain and optimising milk productivity and quality. This presentation will provide insights into the project and will provide practical learnings when it comes to balance interests between scientific research and commercial interests as well as expert-dynamics when traditional statistician meet machine learning engineers to serve a higher well
With more of the world’s population moving to cities, indoor farms that grow crops near urban areas in a way that is efficient with space and other resources may be an important way forward. Dramatic improvements in crop yield and operational efficiency can be made by using data (collected for instance by sensors or cameras) to build an increasingly smart plant factory. This plant factory allows us to control the environment for crops to grow more, better-tasting crops in a way that is reproducible. By iteratively automating processes in the farm, we ensure that this can be done in a way that scales to the levels of production needed. Creating data products that allow us to automate does come with some challenges. One such challenge is balancing the need to develop temporary solutions that provide value early with the risk of enshrining existing workarounds and creating tech debt. In this case study, I will discuss the iterative process we use to automate and improve decisions around the farm, with a focus on operations.
Blockchain-backed analytics (BBA) is a scientific concept to transparently document the lineage and linkage of the three major components of a data-driven project: data, model & result. The approach enables stakeholders of data science projects to track and trace data, trained/applied models and modelling results without the need of trust validation of escrow systems or any other third party. This talk covers the theoretical concept of BBA and showcases a generic appliance. Participants will learn how to design blockchain-backed analytics solution for e.g. situations in which industrial partners share and distribute data, models and results in an untrusted setting.
As with any innovative technology, distinguishing between hype and reality in Predictive Analytics is rather a difficult task. How to predict and measure the future success of a particular solution? Which factors usually hinder its implementation? What are the organizational and business model changes that subsequently arise? IoT Analytics looks at relevant use cases to critically assess Predictive Analytics capabilities not only on the books, but also on the grounds.